There has been a resurgence of AI during the last five to six years.
I use the word resurgence because we have had previous eras when AI was seen as being imminent. Alan Turing devised the eponymous Turing Test in 1950 and brought mainstream attention to the possibility of machines that could think. The Dartmouth Workshop of 1956 was a milestone event that marked the birth of AI when John McCarthy proposed the phrase “Artificial Intelligence” to represent the then burgeoning growth of research into cybernetics, neural networks, and symbolic reasoning.
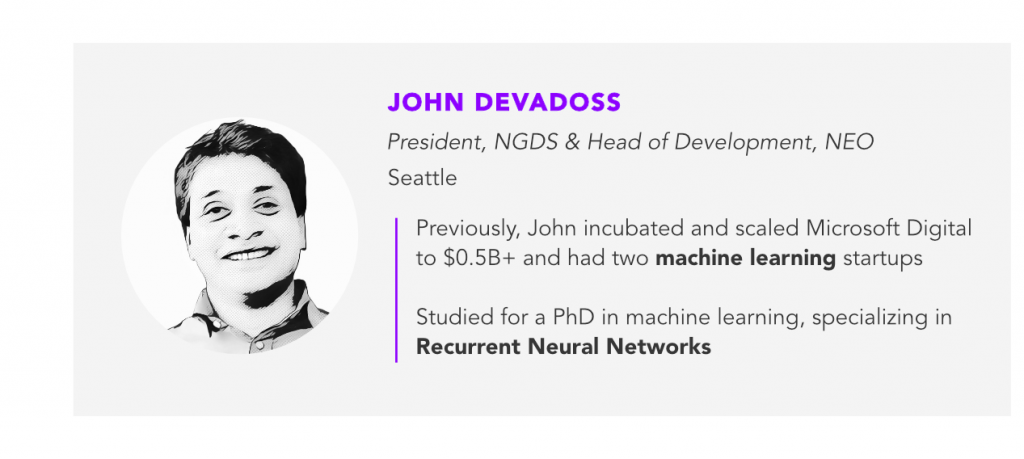
During the ’60s and ’70s there were significant advancements in the areas of natural language processing, machine reasoning, and machine vision. With the advent of the so-called Fifth Generation computer initiatives in Japan in the ’80s there was significant progress in the areas of expert systems, case-based reasoning, and a revival in connectionist neural networks with the invention of back-propagation. Machine learning gained momentum in the 90s with a shift away from earlier symbolic approaches and towards exploiting probability and statistics.
Recently, between the massive investments into both early-stage and mature start-ups; the media heralding a future of killer robots; the marketing blitz from the old-school behemoths including IBM, Microsoft and Google; and the public’s fascination with Siri and Alexa, it appears that AI may finally be here. But, is it here to stay?
Is it Machine Learning? Or is it AI?
Much of what we term AI today results from the application of Machine Learning to extraordinarily large amounts of data. To be precise, it is the application of so-called Deep (Machine) Learning techniques that has enabled the rise of voice-search and voice-activated assistants such as Siri, healthcare innovations in areas such as cancer diagnosis and treatment, face recognition such as AWS Rekognition and the broader areas of image and video analysis and recognition, machine translation including tools like Bing Translator, speech recognition tools and the emergence of the so-called self-driving automobiles and more.
Technically, we should call this the Deep Learning resurgence, and not the AI resurgence.
The history of Deep Learning goes back to 1943 with the creation of the Pitts-McCulloch computer model based on the then understanding of the neural networks of the human brain. The phrase Deep Learning was coined in the late 80s; however, the impact of Deep Learning really took off in the 2000s, and with the so-called Deep Learning revolution starting in 2012 the computing industry was truly disrupted. In March 2019, Yoshua Bengio, Geoffrey Hinton and Yann LeCun were given the Turing Award for their breakthrough research that has taken Deep Learning mainstream.
In simple terms, Deep Learning is a Machine Learning technique that teaches computers to do what comes naturally to humans i.e. learn from examples. With Deep Learning, a computer model learns to perform classification tasks directly from images, text, or sound. The Deep Learning models are trained (both supervised and unsupervised) by using a large set of labeled data and neural network architectures that contain multiple layers of software modeling the behavior of human neurons.
Machine Learning’s Achilles Heel Today
In order to train a Deep (Machine) Learning model today, there are two techniques that while effective today will eventually become the bane of AI.
First, conventional learning approaches use a method requiring the training data to be centrally aggregated on a single system (or vendor datacenter). Google, Amazon, Microsoft, Apple and Facebook aggregate extraordinarily large amounts of user data and store them in their systems. Subsequently, either in a one-time or continuous manner they run their algorithms to mine and to build the resulting Deep Learning models. It will be apparent to the reader that this approach is privacy intrusive. With, and often, without the user’s permission these systems are exploiting sensitive private data in order to build their AI applications.
Second, and equally problematic is that the centralized approach is typically dependent one on vendor i.e. the vendor’s choice of algorithm, their implementation mechanism (language, library, tools), their preferred hardware (in-house, external, dependency on chip manufacturer etc.), their datacenter architecture, their personnel (risk of being compromised, bribed etc.), and their choice of tools to surface the results of the training algorithms. In computer science parlance, the single-vendor implementation is subject to what is known as the Byzantine Fault Tolerance problem.
Either of these techniques on their own is a fatal challenge to the longevity of today’s AI applications. Together, however, they are AI’s Achilles Heel. If the industry does not address them, today’s AI resurgence is bound to once again flame out.
Blockchain + Machine Learning
Blockchain platforms have led to incredible advances in the design and development of decentralized applications and systems and have been applied to domains ranging from cryptocurrencies to enterprise supply chains.
More importantly there are two capabilities that blockchains enable due to their inherent decentralized implementation.
First, blockchains provide the ability for users to be in control of their data and to decide when, where, to whom, and for how long to provide access to their data i.e. blockchains are the anti-thesis of systems that intrinsically and automatically exploit the user’s private data. Further, with the advent of Zero-knowledge proofs, blockchains now have the ability to reveal nothing about a transaction except that is valid.
Second, blockchains are designed without a central authority or system. Therefore, in order to achieve agreement on both data and transactions, blockchains use a variety of fault-tolerant consensus algorithms. While there are an assortment of consensus algorithms, all of them share similar characteristics with respect to achieve agreement across a decentralized set of nodes (or systems). In particular, a variant called Byzantine Consensus addresses the Byzantine Fault Tolerance problem referred to earlier. Blockchains enable the development of AI applications that are not reliant on a single-vendor implementation with all of their concomitant risks and faults.
Together, these two critical capabilities have the potential to enable today’s Machine Learning implementations to address their Achilles Heel and to enable AI applications that are both not privacy intrusive and not susceptible to the single-vendor Byzantine Faults.
What is next
Blockchain + Machine Learning opens up a disruptive new approach to take AI mainstream, whilst safeguarding user privacy and ensuring vendor-neutral applications that mitigate risk.
Extraordinarily large amounts of data are generated by consumers and their devices during their lifetime. This data contains valuable information about users and their behavior: what restaurants they frequent, what websites they visit, what locations they like to travel to, what social media apps they use, what videos they watch etc. This data has become the cornerstone to building effective deep learning models to deliver personalized services to maximally enhance user experiences (ala Siri for example). Blockchains provide a unique alternative to build such personalized models without intruding on users’ privacy.
It is critical that the industry at large address put user privacy above any commercial interests and use blockchain capabilities to build the machine learning models that enable their AI applications.
Applications such as face recognition are becoming widely used (including by government agencies); the risks due to false positives and false negatives resulting from a swathe of issues when machine learning models are tied to a single vendor are far too severe to continue to ignore. For context, avionics systems such as those in Boeing and Airbus planes have been designed to be Byzantine-Fault Tolerant for decades. When tools such as AWS Rekognition are used by immigration services and by border patrol agencies, it is paramount that the industry and regulatory policy agencies re-consider the primitive nature of today’s AI applications (in relation to avionics systems for example).
It is imperative that we move away from single-vendor implementations and their associated risks towards decentralized Deep Learning implementations, using computing resources (algorithms, languages, hardware et al) that span multiple suppliers and vendors. The future is decentralized. And AI is no exception. The alternative is yet another AI winter.