Wash trading is not a new word for people in the financial world. You probably have heard from friends that cryptocurrencies are highly “washed” and round-tripping with the same buy and systematically sell price. Since you are familiar with this term, let me tell you the NFT market has similar issues with wash trading.
In a nutshell, wash trading makes it difficult for non-fungible token enthusiasts to gauge genuine market interest in NFT collections. It also inflates and skews the amount of trading in marketplaces, misleading analysts about what’s going on on trading platforms.
All in all, NFT wash trading is one of the biggest impediments to accurately evaluating projects and assets in the NFT industry, which includes NFT collections, NFT tertiary tokens (think $X2Y2 and $LOOKS) and the studios and developers who bring products to market.
Using Footprint Analytics’ data set to detect and filter wash trading, let us take a closer look at how wash traders operate and how on-chain data could be analyzed to detect suspicious activity.
What is wash trading?
Wash trading is a form of market manipulation where an investor simultaneously sells and buys the same financial instruments to create misleading, artificial activity in the marketplace.
In terms of NFTs, wash trading occurs when the same user is behind both sides of an NFT transaction. It means that both the seller and buyer address is actually owned by the same person. At the moment, wash trading is very common in NFT markets, which are not subject to government regulation or supervision, unlike traditional securities.
Why do people wash trade NFTs?
There are two main motives behind wash trading in the NFT space.
Type 1: To earn platform rewards
Some NFT marketplaces, like X2Y2, reward active users by giving them returns (in the form of the protocol’s token) based on their trading volume. Wash traders take advantage of this and maximize their rewards by generating unrealistically large amounts of trading volume. In turn, this can easily deceive users who want to analyze NFT collections or marketplaces in terms of liquidity and volume.
Type 2: To create an appearance of value or liquidity
To create a false sense of liquidity and an inflated value of a specific NFT collection or asset, some unscrupulous creators turn to wash trading to deceive buyers. They profit when genuine buyers are tricked into buying an NFT from them at a pumped-up price. This type of wash trader hides their activities with new wallet addresses that are self-funded from central exchange wallets. This type of wash trading generates a relatively small volume, which is not as disruptive to the market as Type 1 wash trading.
How is wash trading done?
Due to Type 1 wash trading transactions’ disruptiveness to NFT transaction data, Footprint Analytics aimed to filter them out as much as possible. To understand this type of wash trading, we have to understand the token reward system of X2Y2 and LooksRare. In simple terms, X2Y2 and LooksRare distribute tokens daily to both sellers and buyers based on the address’s trading volume as a portion of the marketplace platform’s daily total volume. Token rewards are fixed daily, so wash traders can wash trade and earn reward tokens repeatedly when the daily distribution resets.
Figure 1 shows an example of wash trading activities on the X2Y2 marketplace— the NFT collection is Dreadfulz.
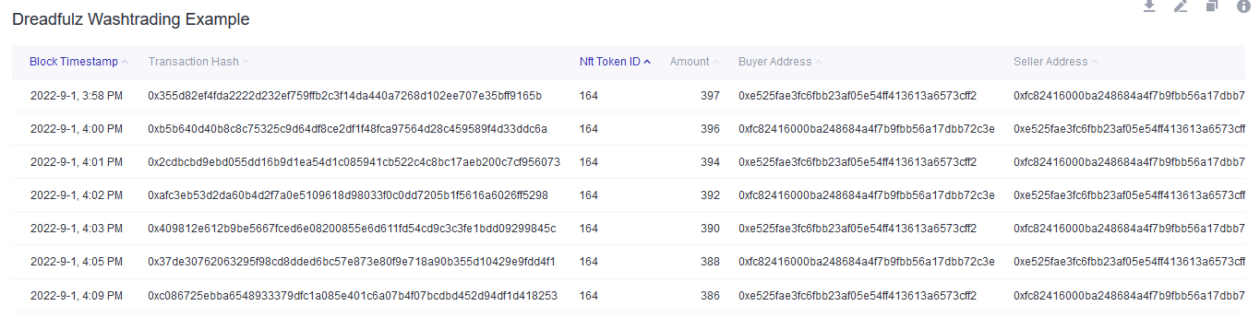
As we can see from the figure above, the same NFT (ID 164) was bought back and forth between the same two wallets several times in a day with 300+ ETH sale prices per transaction. On Sept. 1, 2022, these two addresses traded 19 times, generating 7228 ETH in volume and paying 36.14 ETH in X2Y2 platform fees. Keep in mind that the royalty fee rate for Dreadfulz was not set on X2Y2; therefore, no creator fees were paid. Wash traders will choose collections with 0% creator fees to minimize their wash trading costs.
How to detect wash trading
I have looked at how a few analytics platforms, including Footprint Analytics, do their detection and followed their logic. Their methodologies are somewhat similar, to be honest. Along with my own knowledge and analysis, here is a checklist of suspicious data and activity that should trigger any prospective NFT buyer’s alarm bells:
- A particular NFT is traded by the same address more than X times a day while the rest of the collection remains untouched.
- The same address is trading the same NFT in a high-frequency manner.
- A collection of NFT goes into a self-selling in a high-frequency manner when there is no marketing or promotion backing the sale.
- The average historical price transacted is X times higher on marketplace A vs. B.
- The sale price of an NFT is transacted X times higher than the lowest-priced NFT available for sale.
- The same wallet addresses funding all the suspicious wallets that buy and sell the NFTs.
- An abnormal high trading volume on a constant basis.
The above assumptions are not perfect, and I hope to work with researchers on developing a more comprehensive scorecard to determine NFT trends and behaviors more effectively. The ability to trace multiple wallets over time to identify various levels of relationships would be vital too.
How wash-traded are the top NFT collections?
In Figure 2, Footprint Analytics applied their detection rules to the collections with the most trading volume on X2Y2 and LooksRare.
Based on their rules, they have detected that 95% or more of the trading volume of these collections is wash trading transactions. Wash trading makes up an extremely high percentage of trading volume for these collections, which paints a misleading picture of the collections’ historical volume and sale activities. You can review all the transactions they have filtered at ud_suspicous_txn dataset on their website.
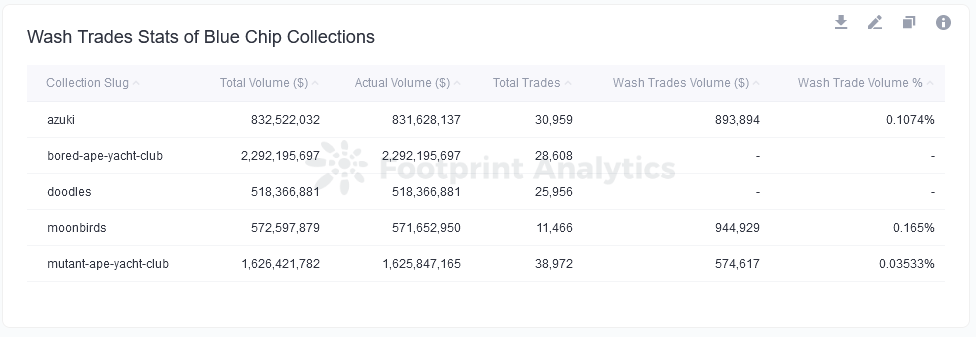
For Footprint Analytics to ensure their rules are working as intended, they have applied them to blue chip collections that are not subjected to wash trading activities in Figure 3. You can view the ud_suspicious_txn_bluechip_collections dataset and review the filtered transactions.
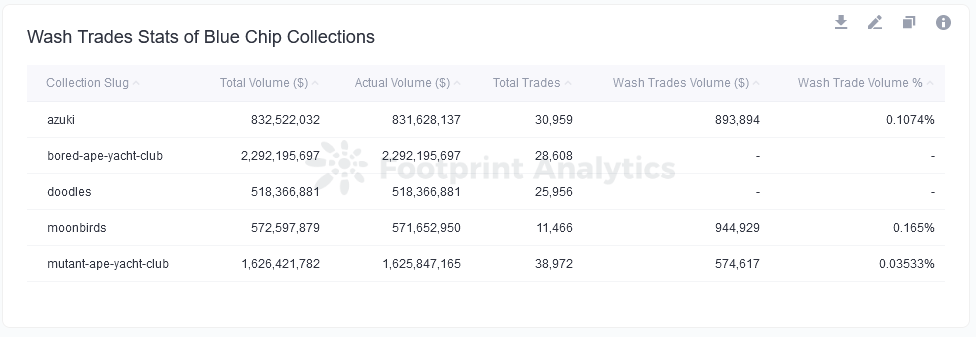
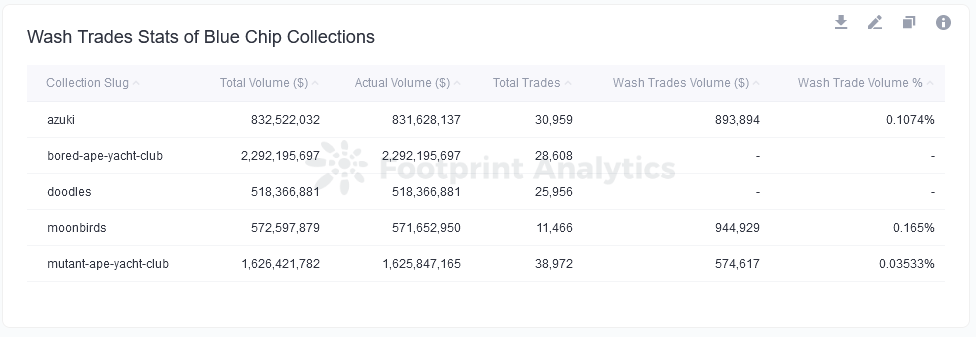
Figure 4 indicates that 94.71% and 81.04% of the trading volume on LooksRare and X2Y2 are wash trading transactions, which appears consistent with the marketplace statistics, as shown in Figure 5. We can see from the unfiltered data that the average price per transaction on Looksrare almost reaches US$85,000, which is around 90 times the average price of OpenSea and unrealistically expensive.
You can view the ud_suspicious_txn_x2_looks dataset and review the filtered transactions for X2Y2 and Looksrare marketplaces, as shown in Figure 4.
Final takeaways
Looking at the monthly trading statistics of the NFT market since January 2022 in Figure 6, we can see that wash trading volume makes up more than 50% of total volume almost every month. Even though total volume is down by a substantial amount from January highs, the percentage of wash trading volume in the NFT market remains similar every month. This underscores how disruptive wash trading is to having accurate NFT transaction data and the importance of filtering out wash trading for any meaningful NFT data analysis.