Between October 2015 and January 2018, when AlphaGo’s AI first beat a professional human competitor at the game of Go, and after it defeated Ke Jie, the top-ranked player in the world, Artificial Intelligence’s popularity tripled, as measured by Google Trends. AlphaGo brought artificial intelligence (AI) out of computer labs and into the living room. Since then, AI has remained one of the most popular search terms and is four to five times more popular than similar well-known technology terms, such as 5G.
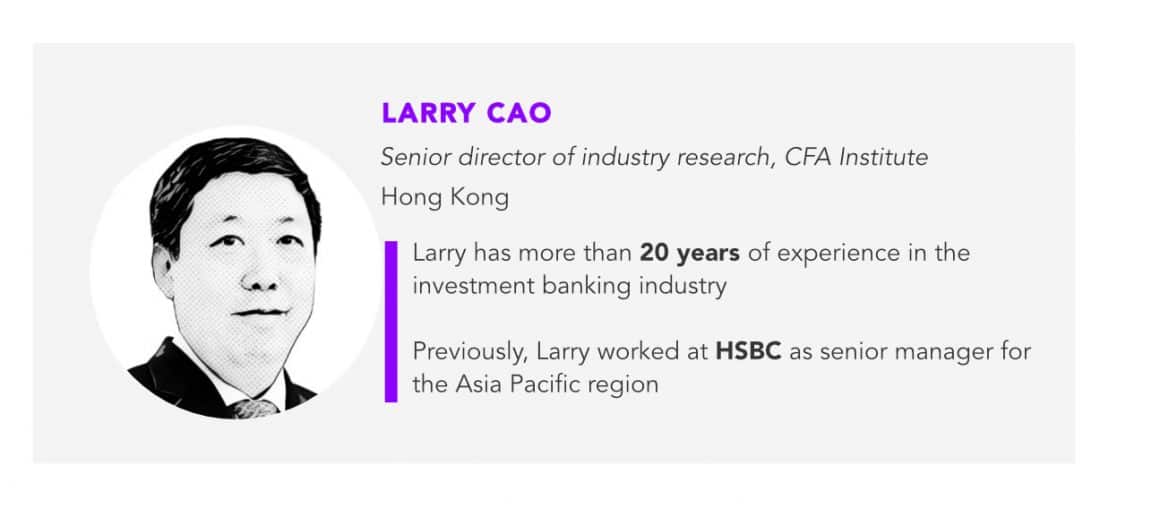
In fact, AI has become such a normal part of life that it sometimes hardly warrants notice.
Investment professionals, however, have been watching the development of AI with a mixture of excitement and anxiety: Can AI beat humans in investing too?
Margaret Franklin, President and CEO of CFA Institute recently said, “We’re at a point now when having well-educated, ethically-centered investment professionals that pursue excellence is critical.” We believe understanding AI and big data’s role in investments and being able to work with technology professionals who specializes in the area is increasingly becoming part of the critical skill set that helps investment professionals excel.
In a recent CFA Institute report, “AI Pioneers in Investment Management,” we talk about the pros and cons of AI. Let me break down some of the report’s major themes:
What can AI and big data do?
AI and big data are definitely not to be feared – they are simply enabling-technologies. Together, with human input, they help us accomplish two things:
- Process new data that we did not have access to or couldn’t process before.
- Process data in ways that we weren’t able to before.
Thanks to advances in natural language processing (NLP), computer vision, and voice recognition, we can now sort and analyze more and more text, imagery, and spoken language through automation. AI programs in these areas have already outperformed the average human.
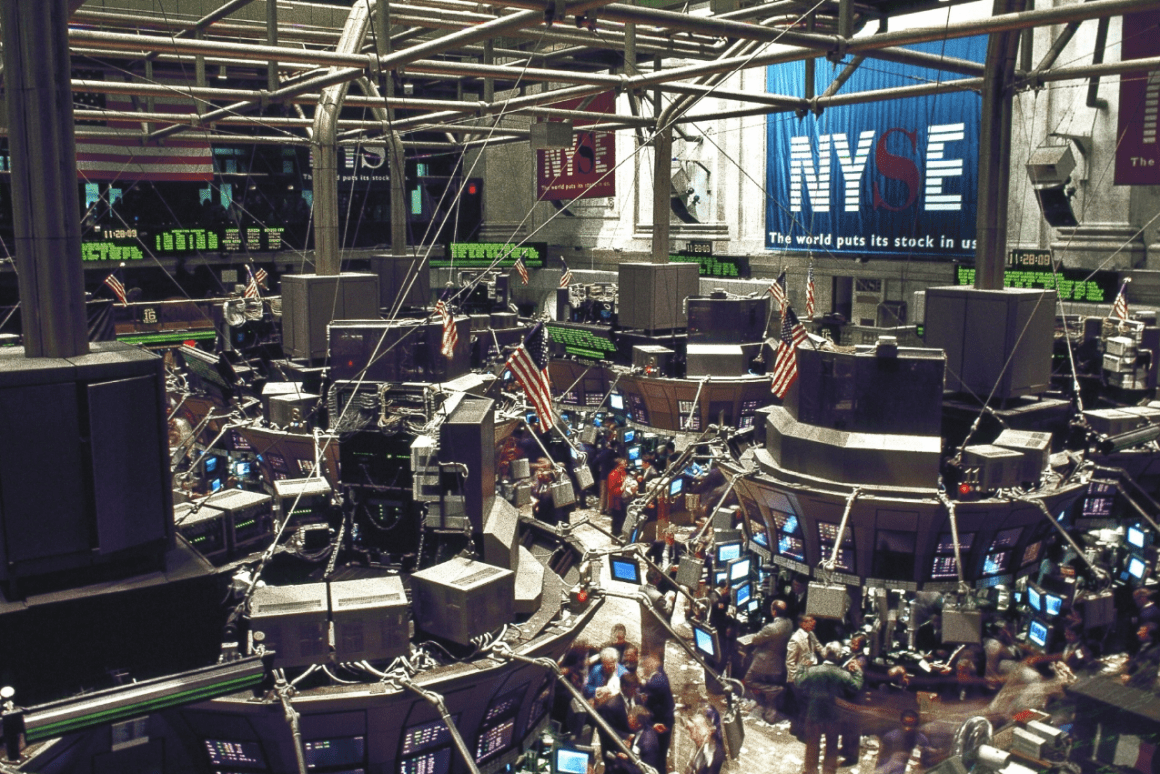
So what can we extrapolate from these developments? That many repetitive and rudimentary tasks — transcription, for example — will increasingly be handled by AI programs.
Big data’s popularity is very much a function of these advances and their expected evolution. AI programs target what’s called unstructured data — social media postings, depersonalized credit card transactions, and satellite imagery, for example — that mainstream analysts rarely used before. This new, alternative data forms much of the new frontier in investment management.
By harnessing advances in machine learning and deep learning, we can find new and more accurate relationships from this data. Much of today’s data analysis still relies on linear programming techniques that place constraints on the variables and their assumed relationships. Machine learning and deep learning have the potential to remove these barriers in many cases.
What can AI and big data do in investing?
AI and big data represent the future of investing. Their broad application is likely to usher in perhaps the most significant change in the history of the investment industry.
With AI and big data:
- Analysts will be able to perform more thorough analysis.
- Portfolio managers will make better informed decisions.
We now not only have access to more and different varieties of information, but also timelier — even real-time — information.
For example, in the old days, if we wanted to independently verify a store’s performance, we might sit in the parking lot and monitor car and foot traffic. In some ways, that approach went too far. In others, it didn’t go far enough. After all, we can only sit in so many parking lots. But big data gives us efficient ways to maximize first-hand data. Rather than staking out car parks, we can buy satellite imagery of lots of store parking lots — indeed, as many as we can afford.
While Tesla’s production information may not be available until its official release, we can estimate staffing levels based on publicly available cell phone data. In fact, that’s precisely what Thasos Group did. By gauging the number of cell phones present near Tesla’s plant, they independently verified that Tesla was running around the clock with three full shifts.
Elsewhere, analysts at Goldman Sachs overlaid publicly available labor information on top of the geometric data of production sites to estimate the market power of manufacturers in aggregate.
Add machine learning and deep learning to big data, and we can now crunch the data in countless new ways. This has vastly expanded the applications of traditional quant methods. We can feed the model input, and the model gives us an output.

Of course, we need to set the parameters properly, but the process makes it possible to capture relationships that might previously have been unknowable. On the downside, there can be a problematic black box effect: The analysis may not yield a true window into the relationships between the input and the output.
See related article: A.I. has fallen short of its promise. But quantum computing can change how machines ‘think’
How should investment professionals respond?
Having read all that, should we now go freshen up our computer programming skills? It would be commendable. But we’re probably better off prioritizing two things:
- Taking our investment skills up a notch.
- Developing a sufficiently broad knowledge base to work well with colleagues/collaborators in technology.
Why? Because the successful investment professionals and teams of the future will be strong in both artificial intelligence and human intelligence. These teams will have both an investment and technology function as well as an innovation function.
The world is getting increasingly complex and specialized. The days of multi-talented operators who do everything on their own are largely over. The expectation for future investment professionals is that they will need T-shaped skills — specialized investment knowledge along with enough technology and “soft” skills to work with the data scientists on their teams. Tech professionals on the investment team will also need to know about investing.
Of course, if you’re the rare talent with sophisticated knowledge of both investments and technology, more power to you. Just remember that you’ll need to spend twice as much effort recharging yourself with continuous professional development.
The main takeaway is clear: AI will transform investment management, but it is not the mass extinction event for human investment managers that many fear. Rather, those investment teams that successfully adapt to the evolving landscape will persevere. Those that don’t will render themselves obsolete.
The future is here. And it is in our hands.