Bias in machine learning applications has been a recurring and controversial topic as growing use of big data exposes consumers to its flaws. Forkast.News Editor-in-Chief Angie Lau has an in-depth discussion with NEO Head of Development John deVadoss on how blockchain and decentralization can help solve the issue of bias and centralization in machine learning and artificial intelligence.
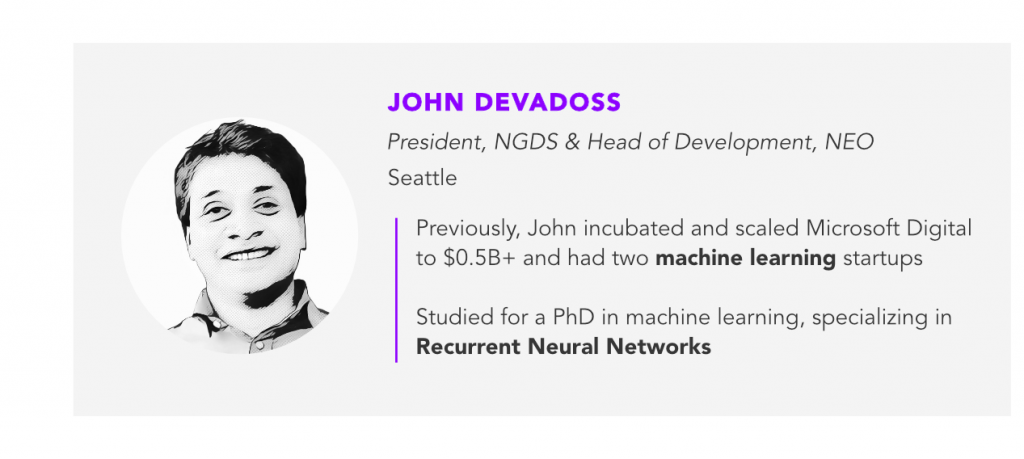
Key Highlights
- I think the fundamental issue here is this inability to parse open within the system and say what exactly is happening, and this is perpetuating this unfortunate challenge of this illusion of AI systems, and AI will take over the world and so on and so forth.
- So much of what AI and machine learning is doing these days is what I call a surveillance machine learning. So what does it mean? It means that your data and my data, the data of the common man and woman on the street is being used is being exploited by these centralized vendors. They are taking your data and our data. And we have no ability to decide why, when? For how long? They have access, so basically, we are at the mercy of these centralized systems.
- Some people use the term decentralized AI. We have to design these systems in a decentralized fashion. And of course, as you very well know, blockchain platforms are the way to go. However, the fact that we today have these extremely primitive systems and we just blame the algorithm, I think is just completely unacceptable.
- I believe that you ought to be compensated for giving access to your data. Today we have this conception that basically it’s for free. It’s not. I mean, it’s your data, it is my data. And so if you want to give access for a certain amount of time to a certain vendor, the crypto economic protocols inside blockchain systems give us the ability to do so.
Listen to the Podcast
deVadoss explains how the data being collected en masse by multinational conglomerates such as Facebook, Google, and Amazon is being used in ways that is to the detriment of individuals. The phenomenon, known as “surveillance capitalism”, is a term coined by Harvard scholar Shoshana Zuboff to describe the commodification of personal information. In her book, The Age of Surveillance Capitalism, Zuboff elaborates on the concept, adding that corporations will increasingly use technology like AI to predict user behaviors.
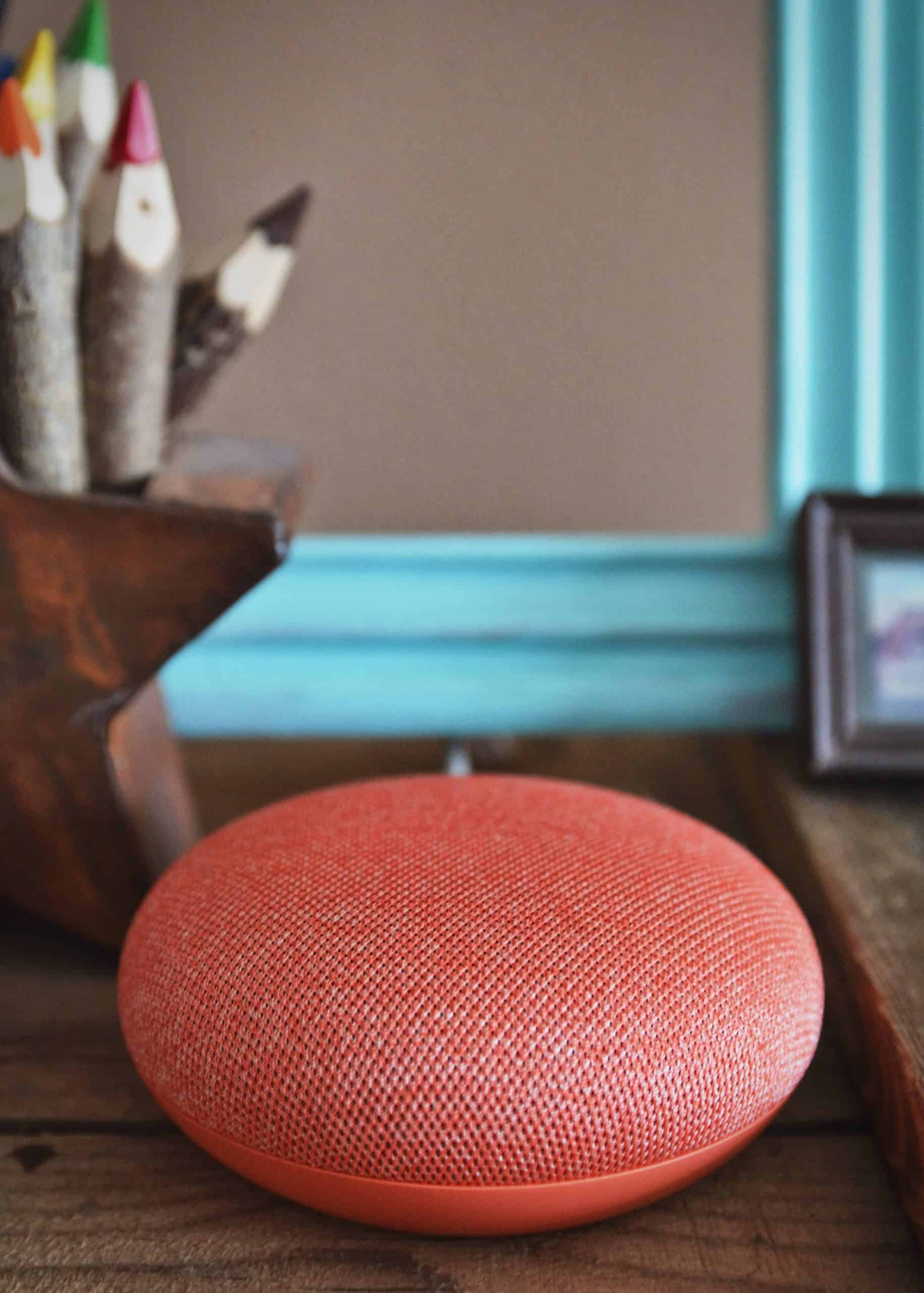
Zuboff argues that AI-enabled communication tools associated with apps, from smart speakers to diet apps, rely on vast quantities of user data which are then fed to machine learning algorithms to predict what individuals or groups of people may want to purchase.
deVadoss says that one way to address this issue in the future is to apply the concept of decentralization and blockchain to the way we share our data. He says that not only would that allow individuals to take ownership of their data, but it might also help solve biased outcomes from machine learning.
There have been a number of cases in which products using machine learning have been shown to have a gender bias. Moreover, US hospitals were recently put under scrutiny after it was discovered that a widely used algorithm was discriminating against millions of patients of certain racial groups.
According to deVadoss, such bias can be mitigated against by using a “byzantine” approach to machine learning. Currently, centralized databases control a large amount of data, and use algorithms to process results for different applications. However, if a number of algorithms could calculate their own results based on the data and compare their output, it might be a way to avoid mistakes that could be the cause of one flawed calculation.
See related article: Opinion | How Blockchains Save AI
Full Transcript
Angie Lau: Welcome to Word on the Block, the series that takes a deeper dive into the topics we cover right here on Forkast.News. I’m Editor-in-Chief Angie Lau. Increasingly, artificial intelligence is taking on ominous tones machine learning, facial recognition and concerns about bias where human programmers, unconscious or maybe conscious bias is baked into the AI thinking and its increasingly discomforting for a lot of people and a concern. But can blockchain be a savior? Our next guest right now is John deVadoss. He’s head of development at NEO. Previous to that, he’s a founder of two machine learning startups, has a PhD in machine learning specializing in recurrent neural networks. His work now centers around blockchain, but there’s no doubt that emerging technologies are colliding. And John joins us from Seattle. John, welcome.
John deVadoss: Hey Angie, how are you? Thanks for having me on, I appreciate it.
Angie Lau: Absolutely. Let’s dive right into the article that you wrote that we shared on Forkast.News, your opinion piece on how blockchain can save A.I. So as I was preparing for our chat, John, I learned that artificial intelligence was a term coined all the way back in the last century, actually 1956. Based on neural networks research in the 1930s and 40s. I mean that’s the Great Depression period, and the recovery since then. Fast forward to today. Help us understand where we are in the first quarter of this 21st century, almost 100 years into the future. In this stage of A.I. technology, where are we right now?
John deVadoss: Fantastic question, Angie. So the way I would say it is, whilst much of the focus, the marketing and shall I say the hype talks about AI, the reality behind AI is really machine learning, the ability to look at extremely large amounts of data and to mine the underlying patterns behind the data. So much of what we think of as AI, data sets, doing voice recognition or even the so-called self-driving car is just a rapid sequencing of being able to see a pattern to recognize it and then to respond to the pattern. So much like the early movies, if you will, when we went from silent movies to the talkies. The key was to basically play these frames so rapidly that there was the illusion of motion in the screen and that’s how I think of AI So the rapid pace of being able to see, recognize, and respond to a pattern creates this illusion of intelligence.
Now, with that said, I think you said, where are we today? If you look at the history of machine learning and even AI, Angie, there have been these sort of, how do we say it, so-called AI winters. We have had peaks and troughs. And I think a big reason for this has been the expectations, that we have set the expectations too high. And each time as we fail to deliver, we kind of end up in a trough of disillusionment. And once again, the cycle repeats itself. However, there is one thing that is different this time around. The ability to use cloud computing resources very economically, almost very cheaply to be able to mine these patterns is something we could not do in the past ever. And this has been the turning point. The reason for this surge of interest in terms of startups and VCs and certainly the industry buzz is cheap computing, extremely large amounts of data and using this to essentially mine and build these deep learning machine learning models.
Angie Lau: I really appreciated how you really elegantly described what machine learning is in terms of technology moving at such a fast pace. It creates an illusion of intelligence. And yet it’s extraordinarily intimidating. And the illusion of intelligence is also mistaken for intelligence. And those sentient beings being humans are also outsourcing the thinking to what you term is the illusion of intelligence. There is a danger in that. There’s a concern there.
John deVadoss: Oh, absolutely. I mean, I like the way you summarized that. Very beautifully said, you’re spot on, I think, on one side. There is this growing trust, if you will, on this notion of intelligence inside the systems. And certainly on the other side, there is almost this fear, sometimes even a paranoia that the system will take over. And I think you’re right. You nailed it. When you piece open these layers, what you have is not truly, in my opinion, intelligence. It is the illusion of intelligence.
In fact, I joke with people. I tell people, look, it is not AI systems that I worry about. I worry about bad code. I worry about bugs in code because bugs in code are really what cause significant challenges and issues for us in terms of humanity, in terms of our economic system. So there you go. There is certainly some element of the industry hype and the marketing machine kicking in. However, as I said in my article, Angie, I think the fundamental issue here is this inability to parse open within the system and say what exactly is happening and this is perpetuating this unfortunate challenge of this illusion of AI systems, and AI will take over the world and so on and so forth.
Angie Lau: And so where does blockchain come in?
John deVadoss: Fantastic question. So that is what they call the Achilles heel of AI, Angie. So for me, the Achilles heel of AI is centralization. So what do I mean by this? Two things. First of all, you’re pretty familiar with the term, I think it’s been called, what, surveillance capitalism? So much of what AI and machine learning is doing these days is what I call a surveillance machine learning. So what does it mean? It means that your data and my data, the data of the common man and woman on the street is being used is being exploited by these centralized vendors. Again, not to pick on anybody, but certainly, it being Amazon or a Google or a Microsoft or an Apple. They are taking your data and our data. And we have no ability to decide why, when? For how long? They have access, so basically, we are at the mercy of these centralized systems.
Angie Lau: With or without our consent. And mostly it’s without our consent or even without our knowledge.
John deVadoss: Absolutely right. In fact, it is often without our knowledge. But there is a deeper issue. So, again, not to pick any one vendor, but let’s go with Amazon. They have what is called Rekognition, which is used to recognize faces, facial recognition. The challenge is this. When you have commercial and certainly oftentimes even public sector agencies like the customs agency using a single vendor centralized solution. The problem is this. So essentially you have one vendor whose people design the software, the algorithms, the hardware, the chips, the data centers, the personnel who write the code, the people who run the data center. And so you are completely at the mercy of one centralized vendor.
Any compromise happening at any point in this chain essentially opens you up. And so if you think about the implications, for example, if the customs agency were to use a service like this to decide who shall be let in, we should not be let in. You can imagine the potential repercussions. So for me, this is such a primitive view of how we look at machine learning. I argue in about five years or seven years, we will look back and say, how stupid were we? How did we let ourselves be lulled into this sense of security by these vendors to use their systems. And this is where blockchain comes in. Being able to, first of all, to decide when, why, how long these systems have access to our data. Obviously at our control.
And secondly, being able to have multiple vendors, being able to have, let’s say, for example, an Amazon and Azure, a Google or an IBM and so on, and then triangulating across these systems. And as you very well know, this is exactly how consensus works in blockchain systems. We have multiple nodes, we have multiple systems, and together they come to consensus on a decision. That is the right way. That is the only way to do machine learning. Unfortunately, today, we are nowhere close to that execution model. But more egregiously, there is a lack of understanding of the risks of how we do machine learning today.
Angie Lau: Ok. So what you’re saying is actually, if we can apply blockchain architecture, really the philosophy of blockchain, and all of the things that it comes with. The different nodes, the decentralization of the consensus mechanism, the philosophy that really drives the spirit of blockchain, that actually needs to be implemented philosophically into AI thinking as well. Is that what you’re suggesting?
John deVadoss: Absolutely. Spot on. As always, you took my words. Then you had a fantastic summary of this. You’re absolutely right. I think that the philosophy, I think the economic model, and certainly the technology architecture that is behind decentralized blockchains have to be infused into machine learning systems. And unfortunately, though, we are nowhere close to being there, I believe this is the only way. There is a lot of talk about, for example, so-called ethical AI Angie, how do we get that? Well, we will not get anywhere anywhere close to ethical AI if we put ourselves at the mercy of one vendor. It’s going to be their…
Angie Lau: I absolutely agree with this, and in terms of the concern, the bias, when you have one centralized decision-making source and we’re talking about source code here, it’s almost the ultimate word. And if that is in control of one centralized vendor or developer or group of people, suddenly global thinking and implementation of “thinking” or “the illusion of intelligence” is now only derived from a handful of people where in society how we’ve evolved as humans, as societies, as a civilizations is really an amalgam of millions of lives over centuries.
So to all of a sudden compartmentalize that into source code written by a handful of people at one company to then implement to a global system, there’s going to be some tension there. That’s got to be a source of concern. So in terms of blockchain, how does it get worse?
John deVadoss: I mean, look, I think this is a recipe for disaster. You look at, for example, personnel being compromised. How long will it take for us to recognize that? Probably a very long time. And in that time, what is the risk to human life? In terms of the systems we run today. So I think that is a much more deeper issue here then I think unfortunately, the mainstream media understand. I’m very happy to hear you call this out. I mean, I’m so happy to hear you summarize this so eloquently.
Angie Lau: That’s what we do at Forkast.News, or at least we try. But look, you’re bringing attention to something that increasingly more and more people must understand. It really is integral. And it’s bubbling up. You’re hearing Steve Wozniak and his wife both, calling out Apple for giving him double the credit on his Apple account than his wife, and they share the exact bank account.
They share this exact salary information. They they share their wealth. And yet she, the only apparent difference is that she’s female and he’s male. And so there is increasingly these concerns. How does blockchain besides philosophy, though, when it comes back to data and deep learning and aggregating this private data that we should have control of but don’t, how can blockchain play a role in that with technology?
John deVadoss: Absolutely. Again, a very relevant question. So I think you’re right, that was very much in the news the last couple of weeks with Woz and obviously his wife. And so the way I see it, Angie, is this: When you have a single vendor, a single system and the so-called sort of relying on a single algorithm, the algorithm’s biases inherently come through. And again, not to obviously impute or put any particular blame on the people who built the system. The fact is these systems are inherently biased. So what do you do?
You say, look, we will have three, maybe four different algorithms for different systems. And ideally, each of these systems have different software vendors, different hardware vendors, different data centers, obviously different sets of people and personnel. And then you triangulate and say, OK, so across these four different systems, what is the consensus? Do we say in this case Woz gets so much but that his wife doesn’t? If you put yourself at the mercy of one system, one algorithm, this is exactly what happens. I’ll give you an example.
Jet planes, the Boeing planes, Airbus, for decades have used a byzantine approach for their sensors. What does it mean? For example, if you’re looking to check the altitude, typically they have three, often four different systems and the systems come from different chip vendors and different software and tools vendors and so on. The idea being this; that all these three or four different systems should have consensus before you relay the information on to the pilot and so on and so forth. In fact, some people argue the reason for, or at least one of the reasons for the 737 Max challenges is because of cost cutting and the fact that they went away from a byzantine approach to a single system approach. And as we very well know, the loss to life, I mean, obviously the risk in terms of credibility and certainly that the market value of companies like these are at risk.
And so it’s very simple. Some people use the term decentralized AI I’m not a big fan of the phrase, but I guess you could say it that way. We have to design these systems in a decentralized fashion. And of course, as you very well know, blockchain platforms are the way to go. Well, we can decide, I mean, which algorithm for consensus and so on and so forth. However, the fact that we today have these extremely primitive systems and we just blame the algorithm, I think is just completely unacceptable.
Angie Lau: Well, at the end of the day, when it comes to artificial intelligence and really blockchain itself, the data is the vital life force, right? The fuel to the engine. And in this case, proprietary data is power for AI if source data is corrupted, that’s a problem. If suddenly we can reverse, we can create controls of my personal information that I choose to share that then is used. Or maybe I donated or contributed to greater AI understanding. And that machine learning then comes from that, based on a decentralized model where it is not one source code, it is multiple source codes. It is multiple points of data entry that potentially this illusion of intelligence can become a network of intelligence.
John deVadoss: Very well said. In fact, I would go one more step, Angie, which is I believe that you ought to be compensated for giving access to your data. Today we have this conception that basically it’s for free. It’s not. I mean, it’s your data, it is my data. And so if you want to give access, for a certain amount of time to a certain vendor, the Crypto economic protocols inside blockchain systems give us the ability to do so. So for me, this is a very natural fit where, you should have this mindset of, look, if I give you access to my data, then what is in return, my compensation? And very simply put, on any of the major blockchain platforms, these economic protocols exist. So once again, I see a very natural segue. Well, I think it’s a question of time now before we find this match of blockchain and AI.
Angie Lau: So the term artificial intelligence was coined in 1956. All right, let’s coin a term now, John. Where are we with AI? What is it that we need to know that really encapsulates today’s concerns so that the future doesn’t look as scary as “one corrupted source code”.
John deVadoss: Yes. And that’s a really, really precise, insightful question, Angie. I would say, to boil it down to simple terms, I would prefer if we did not use the phrase AI. I think it just creates, like I said, this illusion, sometimes the hype and certainly sometimes the paranoia as well. For me, it’s machine learning. Being able to have machine systems learn from large amounts of data. What are they learning? They are learning patterns. So you could say, look, it’s pattern-based learning. Now, with that said, if we look at perhaps 2056, which is not too far away, I think you’re spot on, which is this notion of a collective, a decentralized, consensus based set of systems that can mine and learn patterns, I think, is where we end up.
And hopefully along the way, we lose this tagline called AI because frankly, I’ve spent many, many years in academia in this space. And frankly we have no idea how the brain works. For us to have the arrogance to say that somehow we can mimic it, let alone replicate it, I think is the height of arrogance. I’d be much more happier saying that if we can build a collective set of systems, decentralized consensus based with the economic incentives for users to benefit from giving their data, I think we’ll be in a much better place.
Angie Lau: John, thank you so much for sharing your expertise, your experience and your thoughts on the future. Truly, I think you’re an incredible resource and we will absolutely join you again on the next topic right here on Forkast.News. Thanks for joining us, John.
John deVadoss: Thank you very much, Angie you take care. OK. Have a good day.
Angie Lau: Absolutely, and thank you, everyone, for joining us on this latest episode of Word on the Block. I’m Forkast Editor-in-Chief Angie Lau. Until the next time.