A.I. has fallen short of its promise. But quantum computing can change how machines ‘think’
Alternative AI founder Eberhard Schoeneburg speaks with Forkast.News at Hong Kong FinTech Week 2019 about how artificial intelligence should adapt to quantum technology.
There’s a lot of convention behind the term “artificial intelligence,” and potentially that is the problem. Conventional models for AI, which are based on how the human brain might work, are not effective as we still don’t have a definitive understanding of how the brain works, says Eberhard Schoeneburg, founder of Alternative AI.
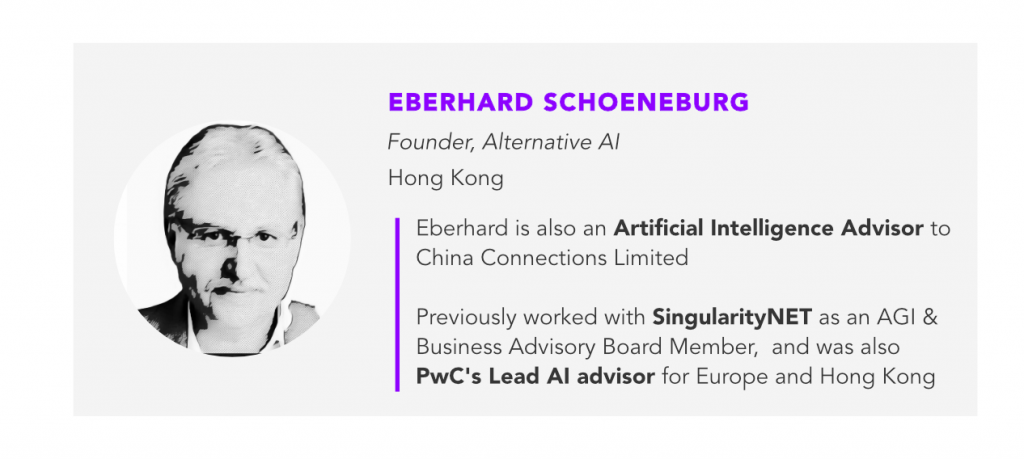
He believes a new way of thinking must be adapted for AI. “Even if you have a very simplified model of the brain, it wouldn’t solve all these issues or all these problems. The key aspect of Alternative AI is to come up with explaining intelligence without referring to brains,” says Schoeneburg.
But quantum processes in nature can be studied for insights to create AI with actual intelligence, known as Artificial General Intelligence (AGI). That may soon become a reality. As Google claims “quantum supremacy” in the developing field of quantum computing, some experts suggest the breakthrough could be a boon to the field of artificial intelligence (AI) and vice-versa.
In a recent interview with MIT Technology Review, Google CEO Sundar Pichai gave credence to AI as it “can accelerate quantum computing and quantum computing can accelerate AI.”
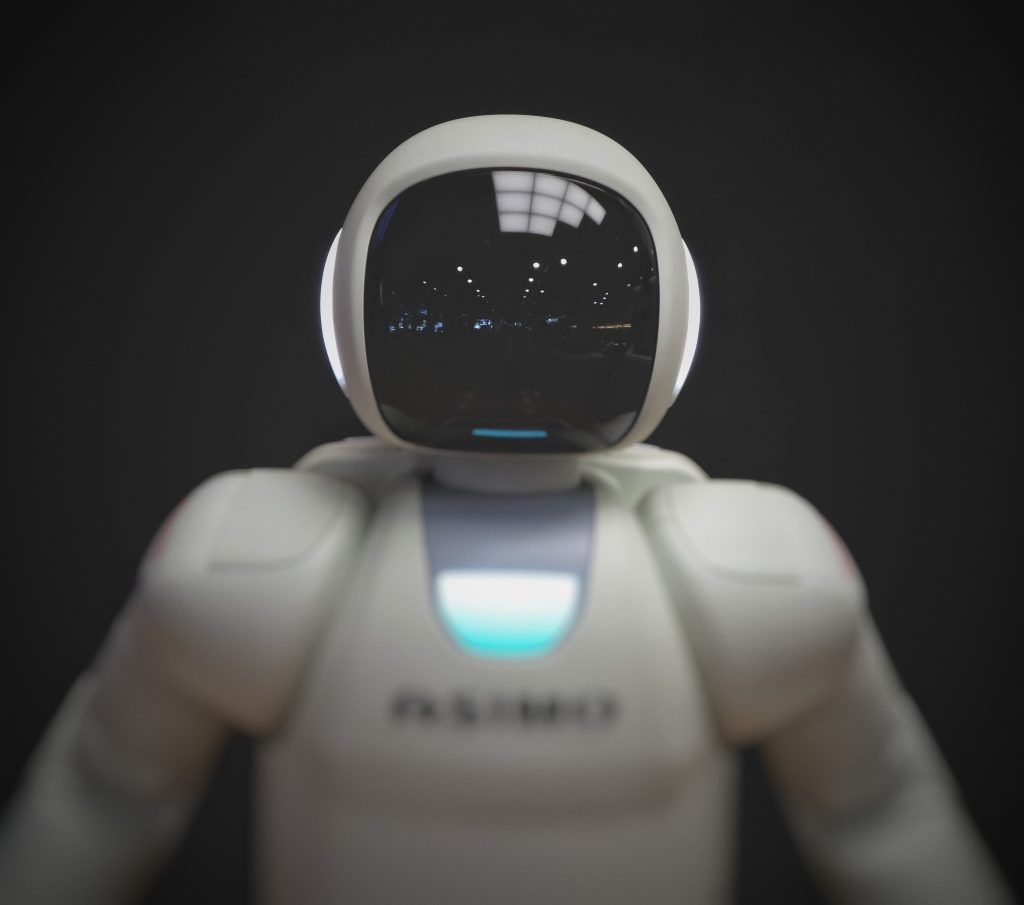
See related article: How blockchain can save A.I.
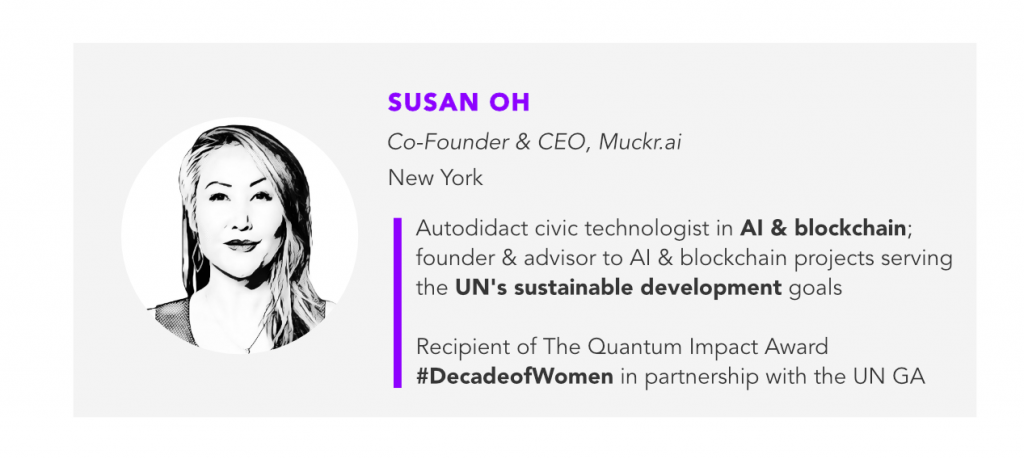
Deep learning methods used in AI currently have narrow use cases which rely on static pattern recognition, while a quantum-based system may be more suited for real life applications, says Schoeneburg.
Nonetheless, other analysts are less bullish on the prospect of quantum computing applications in the short term.
Schoeneburg explains how artificial intelligence should adapt to quantum technology and more. This Forkast.News exclusive brings together two leading voices in artificial intelligence today: Susan Oh, founder of Muckr AI and who also serves as co-chair of AI, Blockchain for Impact for the United Nations General Assembly, sits down with the “Godfather of Alternative AI” Eberhard Schoeneburg and calls out “deep learning” as being too specific to be “intelligent.” To understand the future of AI, one must understand the roots of its past.
Full Transcript
Susan Oh: I have the great honor of sitting down with Eberhard Schoeneburg, who is the godfather of Alternative AI. He’s also the man that gave us [one of the first] chatbots, though he says that he thinks it’s a gimmick and bullsh*t now. So Eberhard, thank you so much for sitting down with me.
I think we both agree that AI has failed to live up to the hype and the promise. I don’t think what people realize is that this is the fifth wave of AI, that people have been working on intelligent computing systems since the 1950s. So if you can, tell us why you think that AI has failed to live up to its promise.
Eberhard Schoeneburg: Yeah, actually, I think the view that AI started in the 1950s is actually an American view. I am German, so for us it started with Leibniz already, in the 17th century. It’s actually very interesting because Leibniz tried to invent a kind of language that is able to represent thoughts that you can compute because he invented also the first mechanical computer, kind of machine to calculate. Now, I think what has happened over the last 60, 70 years, some of it is OK.
Surprisingly, in the beginning, people were trying to solve much more difficult problems than they do now. So what you see now, you see a lot of specialization, very specific kind of applications of AI and that is where the kind of success comes from. But the real problems have not been solved. AI systems are not really smart. They don’t understand what they are doing. They can’t really solve very complicated problems unless are very vertical in very specific areas like games. But on everyday life level, which is much more complicated, there has not been really progress in artificial intelligence in my eyes.
Susan Oh: Is that why you say that we need an alternative? Give us a definition of “Alternative AI”.
Eberhard Schoeneburg: Yeah, that’s exactly why. I was working on a book about computer consciousness. Is it possible for computers to develop consciousness? I ran into this book of Roger Penrose, who was the first one who suggested that to understand how the brain works, you need to understand quantum theory. And I thought, that’s completely nuts, as everybody thought at the time. But then I went deeper into it and I realized that there’s something there that is very interesting.
And then over the last five to 10 years or so, a completely new science has come up called quantum biology, which supports the view that there is quantum processes at the heart of a lot of things in our body and in biology. I thought it’s worth following up on these things and trying to come up with real intelligence in AI systems. And the major difference is that it’s not based on a brain model, what normally AI is, especially the deep learning hype is all about.
We do something that works like the brain and it’s complete nonsense in my eyes because nobody really understands how the brain works. And even if you have a very simplified model of the brain, it wouldn’t solve all these issues or all these problems. So the key aspect of Alternative AI is to come up with explaining intelligence without referring to brains, and that’s what it does.
Susan Oh: As you know, nobody will actually come out and say this, but that deep learning and deep networks and recurrent neural networks, they only work to very specific problems that they don’t even really work all that well. But no one wants to come out and say it. But you have. So what would work better?
Eberhard Schoeneburg: That’s a good question, but very difficult to answer. And nobody knows what would work better. But what is clear now is that people understand that deep learning is good for a static pattern recognition, that you can recognize a pattern. And that is what works in a lot of applications, static patterns. For example, even for self-driving cars, objects do not change so easily. If someone walks across the street, the angle, how you see that person changes, but it’s still the same person. So the person doesn’t suddenly disappear here and appear somewhere else.
So reality behaves non quantum-normally. So that’s why even very simple algorithms work for a lot of very simple cases and in games especially, because the boundaries are very clearly defined. You can’t exactly say when the program played well and when it didn’t because it lost or not. But in life, you cannot say whether a person does well or not so well. It depends, how do you define doing well? It’s not all about making money or about being healthy. It could be any kind of thing. And the codes can change all the time, my codes change I don’t know how many times over my life.
So the boundaries are very, very tricky to catch the goals. The goals are self modifying in all kinds of things. And that’s what makes it so hard. And nobody really knows where it’s going. And the only thing I think that is worthwhile following is to have a much more dynamic view of things, to have a dynamic science system, you know, political systems, attractors, so that the focus on patterns is changing towards a more dynamic vision of the world.
Susan Oh: So isn’t what you’re saying is that in real life, we have much more dynamic systems with a thousand causalities using more fuzzy logic and different conditions all the time. And so that’s why the alternative AI that uses both biomimicry and quantum mechanics is much more suited to what’s real life. Do I have you correct? So what are the use cases and implications of this? I mean, I can see it being used everywhere.
Eberhard Schoeneburg: Yeah. I mean, here and in fintech, I focused on financial applications, obviously. In finance it’s a big issue. In finance, there’s a lot of companies that have a lot of money. They have the resources to hire the brightest people. But all what they do is, they either do quantum, but quantum is in too early stages, quantum computers cannot really solve any serious problem so far. Or the focus on deep learning and related kind of things.
Classical AI, deep learning has been invented in the 80s. So it’s like thirty five years old. It’s crazy. So science has made major progress. So I focus on more dynamic aspects of analyzing financial systems. So the development of attractors and dynamic systems. What I think a very interesting area is, a lot of financial markets, they suddenly collapse. Nobody knows why when it happens. You always come up with an explanation after it happened. You come up with all kinds of reasons why it has happened, but nobody can really predict a collapse of a market. So and why do some markets collapse and why do some markets not collapse? That is a very interesting question.
I believe it’s because the markets are very highly complicated, dynamic systems with a lot of feedback among all the players. And the matter is, are they are stable attractors in it or not? Do the systems settle into a kind of stable states or not? And if they do or if they don’t, what happens if there’s a perturbation? If something comes in your holdings, tickles, jiggles and wiggles around, does it collapse or not? And if you study dynamic systems, that’s the only way to do that– by assimilating these these processes, these feedback loops. There’s not even a mathematics for that.
If you want to do differential equations, the best we can do is handle 3-4 parameters. But the financial market has like millions of parameters, millions. There’s no way of doing it, so you need a smart computer models and dynamic computer models. And I try to work with the most simple models like cellular automata, because the more complex models are, then the more complex the understanding, where you won’t understand it. So if the basic model is at least very simple, there’s ways to understand it. And that’s why I focus it.
Susan Oh: If we can back up a little bit and you can just help people understand what cellular automata is.
Eberhard Schoeneburg: All right. So that has been invented by the pioneer of computing by John von Neumann, a Hungarian polymath. It’s actually very simple. Our skin is actually also a computer. So every skin cell changes its behavior depending on external forces, but mostly depending on what the neighboring cells are doing and what state they are in. So they all influence each other. And this is what the cellular automata does. It’s considered like a grid. You have a plane and you have squares. And each square in the grid is a cell. And it looks at what the neighboring cells doing. And depending on what the neighboring cells are doing, it changes its own activity. And every cell is doing this at the same time. And that creates this crazy feedback loop and dynamic.
Susan Oh: When I was looking at the slime activity and how it invades, one of the most fascinating things that you said that made complete sense was that completely non-intelligent beings or organisms can create intelligent systems when they act together. And that’s relational like that cellular automata that you were describing. Is this how you see our financial systems and our manufacturing systems behaving?
Eberhard Schoeneburg: You mean that they’re not intelligent?
Susan Oh: That you can have non intelligent actors in this phase becoming intelligence through each other’s activities?
Eberhard Schoeneburg: As we humans notice, if you put a bunch of people in a room, the group is not necessarily smarter than the individual. That’s not a necessity, but it could happen. And the best example is not a single person can go to the moon or to Mars. But if we all work together, we can do that. So combining a little bit of intelligence, many, many, many of them could generate something. And that’s the whole idea of the cellular automata as well.
So you hope that when you have a little bit of intelligence here and there and you make them all work together in a very complex dynamic, you generate intelligent behavior. And really that is so fascinating, I don’t know why people have not realized that earlier. Now, when you when you heard this this news about this this slime mold, all of the sudden people realized, whoops, this thing is smart and it has no brain. I mean, it is so obvious. But AI hasn’t gotten to that yet, and think about it, even if you adopt the classical AI model, that the brain is made out of neurons, and it’s smart because all these neurons work together. The neurons have no brains. So at the end of the day, even the classic AI model relies on a model that uses no brain. But now the problem starts.
How can you explain intelligence without the brain? And cooperation is just one aspect, right? There’s a lot of lifeforms that do not cooperate and still are very smart. So the cooperation is one way to be smart, but it’s not all of it. There’s an underlying principle, and that’s what I tried to explain. It’s like the standard model is that it’s based on the microtubules and how smart they are. In a sense, they can compute things by vibrations and stuff like that. And intelligence essentially a resonance of vibrations. It’s like music, more or less.
Susan Oh: And by this you mean that music is formed by spaces in between the notes, correct? And that it’s done frame by frame pieced together and that’s what you’re saying is what our consciousness is, a series of flashes. Correct? So then how would we begin to look at that through on intelligent computing systems?
Eberhard Schoeneburg: It’s a very difficult question whether one should try to emulate that or whether one should come up with a system like the cellular automata that have the same effect, but not really trying to now start building atomic guitars or something like that. I don’t know if it makes sense to model vibrations, but vibrations are a good paradigm because resonance is easy to understand, right? Harmonies are easy to understand. This harmonic behavior is easy to understand. And it’s just a very different kind of paradigm than classical AI is using with the brain, so but it doesn’t necessarily mean it’s the right way to model it.
So I wouldn’t go into modelling vibrations, but try to understand what do the vibrations do? And what does harmony or resonance do to the vibrations? It essentially strengthens the signals in a selective way. It either cancels out the signals or it enhances the signals. Whether those waves are in sync or not. So this is much more important to understand. It’s not how exactly it is done in biology or in physics. But what are the underlying computational principles?
Susan Oh: See, this is what’s so fascinating to me, because as you know, as anyone that works with anything from distributed computing systems or an AI or a blockchain, or IoT, it’s all about inputs and outputs. The noise to signal ratio that you’re talking about. If we can model it through the variance of a resonance or vibrations. You’re saying that we could get a much more accurate picture?
Eberhard Schoeneburg: I don’t know accurate, but we would get an alternative way to analyze the same things and maybe an alternative. That’s why it’s called Alternative AI, maybe an alternative way to generate intelligent behavior.
Susan Oh: What are you working on now? I understand that you are using the cellular automata towards financial markets to modeling financial dynamic financial markets, correct?
Eberhard Schoeneburg: I do like everyone else, I go where the money is. I follow the money, and that is really true. So I used to have all my companies and everything, but I’m too old for that now. I’m just doing advisory services, but it’s mostly insurances, banks, traders and stuff like that. So I focus a lot on financial applications. But the problem for me is that I’m always a little bit ahead of the market. So even if I go to, let’s say, a hedge fund who has done AI with deep learning and whatever, I’m way ahead again. So these people might not like my idea, right? Because what normally happens if I go to a big thing, like a big hedge fund, they have their group. They have hired 20 PhDs from Stanford or whatever. And they think they know all that. And they don’t. They just don’t.
Susan Oh: Well, you know what happens to pioneers, right? They get shot, and then it’s like the third or fourth wave that actually makes money. It’s funny you’re saying that “I go where the money is” for someone that created chatbots in the 1990s when people were saying things like, oh, why would you even want a personal computer?
Eberhard Schoeneburg: I’m not saying I’m going after the money because luckily, I’ve made enough money already. I don’t I don’t need to work anymore, but I’m going where the money is because it means that there’s the people with the pockets to try out something new. So the situation that I usually bump into is people have tried all the stuff with deep learning and so on. They have little bit success here, a little bit success there. But they’re usually frustrated. They run into cul de sacs. It doesn’t go anywhere from there. And I say it’s no surprise, that’s the reason, and maybe you try this.
But then I run into walls often, not always, but often with the people that are already there, because that’s new for them. So they now are in a situation where they are the one-eyed among the blind. They see a little bit. But they can detail all kinds of garbage to their bosses. But now with something new coming, which they don’t understand, now I’m a threat. You know what I mean? And I have been in that situation for the last 30 years of my life. Seriously, they laugh at me all the time, it’s crazy. Luckily, I’m right, but it’s like 20 years after I have done all this stuff. It’s a bit frustrating. But in the end, I am happy that I survived so long that I can see the success now in some stages.
Susan Oh: It’s interesting to me because you created [one of] the first chatbots, but you think it’s a bit of baloney and that it hasn’t actually fulfilled its promise. Well, when you created it, what did you want it to do?
Eberhard Schoeneburg: Actually, again, it was in finance. So I created the first financial robo-advisors and we had big banks, UBS and Credit Suisse, Deutsche Bank, really major clients. But it was exactly like in 2000, 2001, and then the market collapsed just when we got the first installation, like six months later, everything we had… I was in 9/11, I was in downtown New York. You know, it was catastrophic. So everything came to a screeching halt. So we had to start all over again. But I had actually the finance market in mind. So the first application we developed was a retirement planner bot. So the website was actually for Pioneer Funds in the US, it was a website where you had a bot you can talk to and say, look, I’m 63 years old, I have a house, I have three insurances and I want to retire in two years.
What’s the best thing I should do? And at that time there was no speech to text technology, but you had to type everything in. So we developed a system that you could type in, even in Korean, in Japanese, Chinese and everything. But in everyday language, you can just ask, no pre-programmed special kind of phrases. And I was very proud about that. And it was really powerful at that time. We had a whole dialogue management system. So it’s not enough like you have these days with Alexa and Siri and so on where you ask questions like, “where can I get that pizza?” And they have millions of examples like that, and they can train the system on it. This is just horribly dumb. So my bots at that time already were able to have a real dialogue. So you can ask something the bot would ask back. “Oh, do I understand you right? You want this or that?” And then I’d say, no, no, I want that. And they’d say, “okay, I understand. But how about if you consider this?” and so on, like a real dialogue system, and you don’t have this anymore, it has died out.
Susan Oh: So that’s contextual understanding for NLU and NLP, correct?
Eberhard Schoeneburg: Yes, it is.
Susan Oh: And you had this in the 1990s? And then what happened? Did it just plateau for the last 20 years?
Eberhard Schoeneburg: It was much worse. In 2001 I filed a patent for that, natural language communication with computers. It has now been referenced by everyone, hundreds of the largest companies in the world–IBM, everyone. But you know what I did not do? I did not follow up on their patent filing. So it was not granted like three years later. I got all these questions from the patent office. And I just ignored it, I was so busy. I could have been a trillionaire by now.
Susan Oh: Oh, my God. I hate hearing stories like this.
Eberhard Schoeneburg: I defined the state of the art. It was considered in 2001 state of the art. 2001, now we’re at 2019. But it died out, literally. I see it as like classical music. If you look at classical music, like 200 years ago, 300 years ago, Sebastian Bach was the peak of music in my eyes. So complex, so complicated, so wonderful. It’s not there anymore. Today, everybody who can play three riffs on a guitar is considered a genius.
Susan Oh: Not even, we’re talking turntables. Programmable music.
Eberhard Schoeneburg: AI too, in my eyes.
Susan Oh: I actually know one person that’s been working on contextual analysis for NLU/NLP for about 18 years. And she cracks it then we’ll have much more intelligent systems that can interact with human beings. When people tell me that they’re terrified of AI, that AI is going to kill us all, and with AGI coming, I always tell them we’re worried about the wrong things.
Eberhard Schoeneburg: You know Andrew Ng? He said a funny thing that he worries about when we have overpopulation on Mars. I like that one.
Susan Oh: When you’re talking to other technologists and builders and founders, can through for me some of your process for looking at unintended consequences of what you might create and how you go about creating these systems that seem so far left out field that when you explain it, it kind of makes sense. It totally makes sense. You ran us through so many things. But no, it completely makes sense.
Eberhard Schoeneburg: I think the big problem with AGI is that, again, it has this wrong paradigm of the brain. So I’ve been working with SingularityNET, also the whole blockchain team. I was there from the beginning. But I think the focus is wrong. It’s an engineering approach. First of all, I do not think that you can engineer a brain. It’s way too complicated. I think you have to grow it literally like a biological system. You have to plant it and you have to have the mechanism so it can grow intelligence. So it’s a completely different paradigm.
I also think for engineering, it’s just way too complex. It’s not gonna happen. It might happen someday that we have these very smart robots, but using completely different approaches. So that’s why I focus on micro robots, nano robots. I would be very, very happy if we had insect-like intelligent robots that are the same size, that have the same skills like a housefly. It can fly around, has such a tiny little brain and you cannot catch it. Try to catch a fly, you cannot. You have such a big brain and you cannot.
Susan Oh: This is completely relational decision making processes, correct?
Eberhard Schoeneburg: It’s an optimized brain, it’s a brain for a specific purpose. So what I think AGI is doing wrong is it tries to build a general AI that can solve any problem. We cannot solve any problem, I cannot solve any problem. I can solve certain problems. But I cannot solve every given problem, I’m not so smart. And going along that route, trying to build an AI that can solve all kinds of problems, we’ll just end up in a big mess. Cannot solve anything. But if you focus on specific areas that there’s so much intelligence that is needed to solve that problem, like extremely vertical but more real life, not games, not toy models, real life problems try to build real amoeba. That’s a huge problem.
Susan Oh: That’s the problem with object oriented programming. Even when you look at image recognition, you have to define the parameters by which you’re modeling your patterns, and life simply doesn’t work that way. So then, what is your hope then? I mean, you’re always like 20 years ahead of the market, right? What would you like to see happen with the cellular automata and how it’s being used and implemented?
Eberhard Schoeneburg: Yeah, it’s a two sided sword. In one sense I hope for a breakthrough in AI, I hope to get some really smart stuff. But on the other side, it’s a problem how tiny little robots can get out of hand also. You can you can build artificial microbes, and if you cannot control them, they can be anywhere and it can kill the world, literally. That’s also a problem, so as long as you do not understand how to control them, I don’t want these systems to be too successful as well. So I’m working on both ends at the same time.
Susan Oh: Both ends as in how to make it successful, but then how to put in a kill switch?
Eberhard Schoeneburg: Kind of, it should kill itself. That’s the idea.
Susan Oh: Based on utility or specific rules that you put in.
Eberhard Schoeneburg: Biological life does this. A lot of bacteria kill themselves for the purpose of the greater good. They literally commit suicide, and a lot of our cells do that also in our body, we have that all the time. And this mechanism is barely understood. When does it happen? Why does it happen? I mean, why is it kind of clear? Because if there’s too many of certain things, then resources are getting tight and so on and so on. But which cell decides to kill itself, and why don’t other cells kill themselves? And why does it work? How do you know that not all of them should kill themselves all of the sudden like the lemmings do sometimes? It gets out of control, they commit suicide, it’s completely stupid. So but that’s a very, very interesting problem. People think about emulating life. You also have to think about death, how to kill these things, how they want to be killed themselves.
Susan Oh: Isn’t that the first principle of building complex systems is tight iteration loops with tiny parts of the systems that aren’t mission critical…
Eberhard Schoeneburg: Fail-safe systems.
Susan Oh: Fail-safe, and let them let them die and regenerate.
Eberhard Schoeneburg: It’s a given principle in engineering. But in biological systems where everything grows and it’s not controlled by the central unit, that’s a very different thing. It’s much more complicated.
Susan Oh: That takes us to distributed computing systems. Earlier when you’re talking about we were talking about swarm intelligence and fuzzy logic. It almost sounded like the principles behind blockchain and crowd intelligence or crowd-sourcing and open source. Now, as you know, the strength of open source is the weakness of open source. A lot of things that are crowd-created are very difficult to implement at an enterprise level. Is there a way of directing groups or putting in governing systems so that we get the best of both worlds?
Eberhard Schoeneburg: You mean for open source? So you’re not talking about blockchain? I don’t have answers to everything. I think open source is a blessing and it’s a pain too. I mean, the blessing is the openness of the know-how. But I learned computing in the 70s. You know how computing was at the time? I had to stand punch cards. I’m not kidding. And you know what it gives you? It gives you crazy discipline because I had to literally punch cards. One wrong punch and to get the whole thing back two days later with no processing, due to the mistake. Today every idiot can program by cutting and pasting something from some open libraries. They don’t think anymore.
They just cut and paste and try out and they watch YouTube videos, how to program. I’m serious. I was one of the first to develop digital telephone systems, ISDN systems at Siemens was kind of my first job. You know, we had 64 kilobytes for the whole system memory. 64 kilobytes! Today an operating system has 40 [gigabytes] or some shit like that. There’s no thinking anymore about efficiency or anything like that. You just patch stuff together and there’s no consideration anymore about restrictions. So with having an abundance of ideas floating around, it reduces thinking literally. The restriction of resources is gone, so everything is possible. So you might be lucky and come up with some very new creation. But look out. Look what’s happening with startups in AI, how many are worth talking about?
Susan Oh: You know what? I didn’t think anything could have a worse hit rate than like Silicon Valley and the VC model, but then came crypto and of course, AI is somewhere in between that, and they’re all miserable hit rates.
Eberhard Schoeneburg: Yes, I agree. What frustrates me, I know in my past when AI was not in such a boom as it is now, there were these waves. But I usually had to work always on a shoestring budget. We never had money for anything, but we were extremely creative I think, really. I had the best and the brightest and they came because the task was so interesting. Not because we were swimming in money. Everyone goes to Google because they get free health care and shit like that, not because they’re working on interesting problems, because they have colorful offices and slides and stuff like that. That’s the business model of Google. I mean, sorry, it’s just that I cannot understand it.
Susan Oh: I want you to know that hacker groups are alive and well, whether they’re white, black hat, and gray hat. And there are people that just like unsupervised… they’re clustering in their own natural little ways to figure out some problems. And an open source is still very much alive. Which I’m really happy about.
Eberhard Schoeneburg: Yeah, it depends, I’m on both sides. So when when I did the chatbots and we gave this business up, it then moved to, it was stolen actually, moved to Russia and then it popped up as open source. And it’s everywhere now. So part of most of the bots are just my original stuff. So in a sense, it’s good. In a sense, it’s bad. I lost a lot of money. It’s all gone. It’s all public, no way to protect it anymore. And I don’t know, what is the motivation for some one or even for an industry to invent something if it’s not protected anymore? And in my eyes, if you look at all the big companies, the Googles and Facebooks and the IBMs in the world putting out all this stuff in an open source. Trust me, the good stuff, they don’t put in open source. And you don’t see any military systems that are open source. Why not? If it’s so good and it helps everything and it gets the bucks out. Why not put all the military’s stuff in open source?
Susan Oh: That’s never gonna happen.
Eberhard Schoeneburg: And there’s a reason for it. So there’s good and bad sides, too. I’m not 100 percent a fan of open source.
Susan Oh: Why, because of the quality?
Eberhard Schoeneburg: But it’s a big thing, you know? Remember the war between Microsoft in Unix, right? First it was Microsoft. It was a monopoly. There was no one there. Then Unix came. Everybody was laughing about Unix. Now they’re not laughing anymore. Now Microsoft is using Unix. It just completely turned around. But the problem is that… I’m not defending Microsoft. I’m saying if you have a system that you use and it’s Microsoft, you can sue their ass off if something goes wrong. And you know they are taking care of it if something goes wrong. If you have an open source, you have to find someone who is willing to take care of it. Some some hacker somewhere in East Europe there has nothing else to do and just tries to fix the problem. I mean, if you’re a real industry player, you really have to think three times whether you’re gonna use open source or not. If it’s research or close to research, sure, you would use open source because you cannot afford to buy some expensive licences for something.
Susan Oh: That whole liability issue goes to AI as well. Like what if Sofia goes off and kills somebody? Then who’s liable?
Eberhard Schoeneburg: That might be the only good reason why to make it open source.
Susan Oh: I think it could be said that all innovation is a dialogue rather than the lone hero myth. We walk in the steps of pioneers who were who were shot and lost their stuff and we’re able to build and continue to build. So what is your greatest wish now for your work?
Eberhard Schoeneburg: I try to have some decent success and I still haven’t figured out a lot of things. I’m 63 years old, I learn something new every single day. And I hope to continue doing that. I might go back to studying at university and stop all the business work. I can afford it, so I have no specific goal. I just try to learn and try to understand these things. I try to understand what intelligence is and how it works, and hopefully I get closer to it in my lifetime.
Susan Oh: It’s very inspiring. Thank you so much.